The Bias from Misspecification of Control Variables as Linear
DownloadWe characterize the asymptotic bias that arises in ordinary least squares regression when control variables have nonlinear effects on an outcome variable, but are assumed to enter the regression equation linearly. We show that this bias can be larger than that from omitting such variables altogether, or may vanish entirely despite the nonlinearity. We find that under a natural assumption an upper bound to the magnitude of the bias may be estimated from the data, and consider examples of the bias through Monte Carlo simulations.
Authors
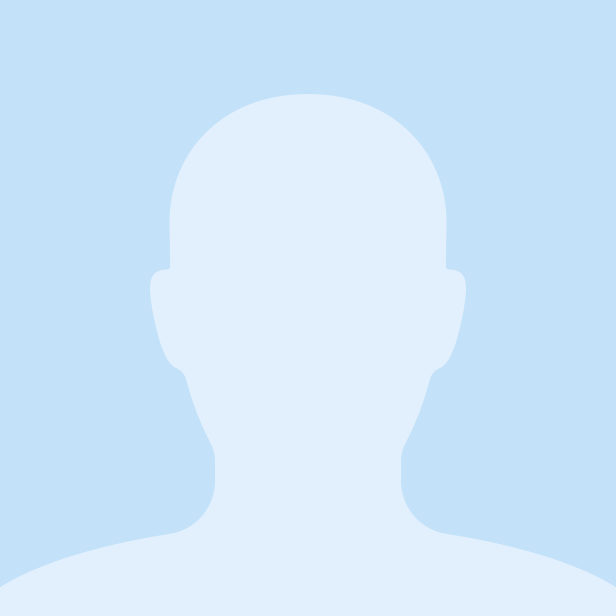
Leonard Goff