Machine Learning Predicts Which Rivers, Streams, and Wetlands the Clean Water Act Regulates
RFF University Fellow Hannah Druckenmiller coauthored this study, which used a deep learning model to find that Supreme Court and White House rules offer dramatically different Clean Water Act regulation.
Editor’s Summary
The Clean Water Act is a defining piece of environmental legislation in the US, but the waters that it protects from pollution have never been clearly defined. Greenhill et al. developed a machine learning model that uses geospatial data to predict which waters are covered by the Clean Water Act and trained and tested the model with jurisdictional determinations from the US Army Corps of Engineers. This work provides an estimate of the extent of protected waterways, as well as an understanding of the effects of Supreme Court and White House rules that have reinterpreted or changed the regulation. For a subset of sites with high predictive accuracy, their model can also act as decision support tool to expedite permitting. —Bianca Lopez
Abstract
We assess which waters the Clean Water Act protects and how Supreme Court and White House rules change this regulation. We train a deep learning model using aerial imagery and geophysical data to predict 150,000 jurisdictional determinations from the Army Corps of Engineers, each deciding regulation for one water resource. Under a 2006 Supreme Court ruling, the Clean Water Act protects two-thirds of US streams and more than half of wetlands; under a 2020 White House rule, it protects less than half of streams and a fourth of wetlands, implying deregulation of 690,000 stream miles, 35 million wetland acres, and 30% of waters around drinking-water sources. Our framework can support permitting, policy design, and use of machine learning in regulatory implementation problems.
Authors
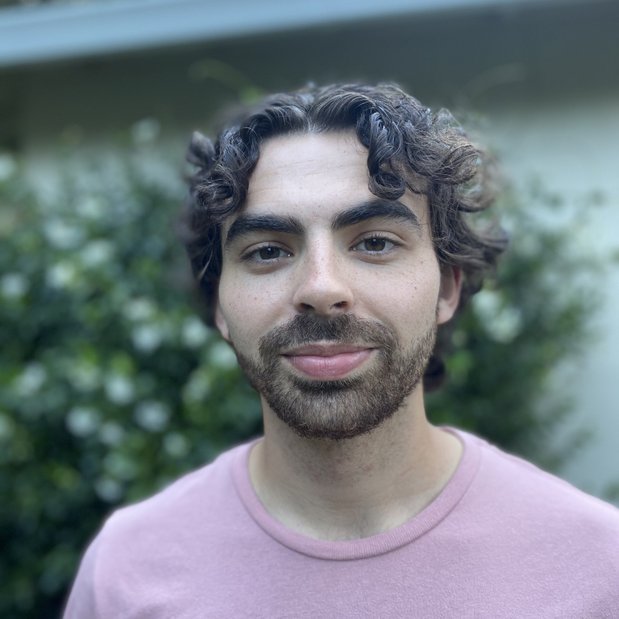
Simon Greenhill
University of California, Berkeley
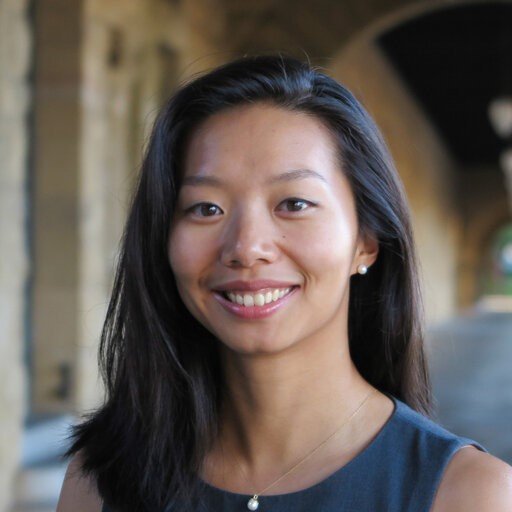
Sherrie Wang
University of California, Berkeley
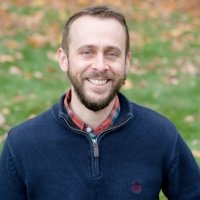
David A. Keiser
University of Massachusetts, Amherst
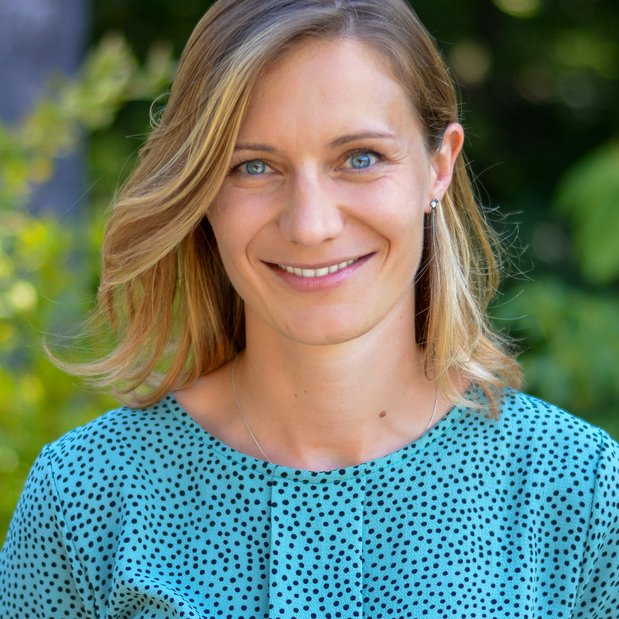
Manuela Girotto
University of California, Berkeley
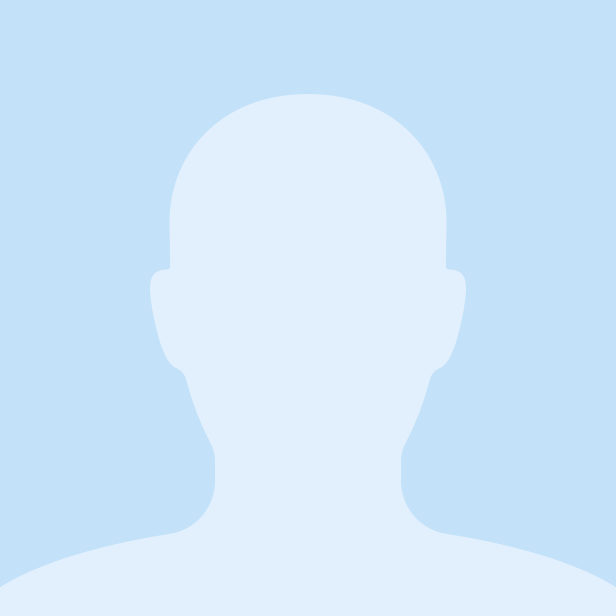
Jason K. Moore
US Department of Energy
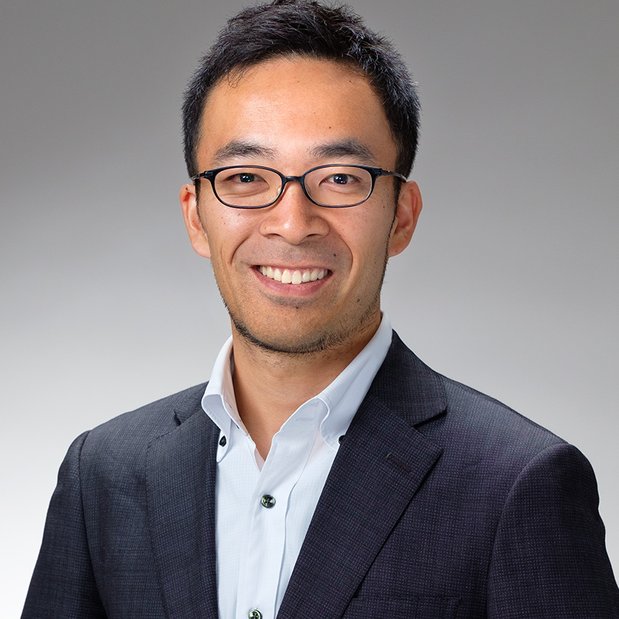
Nobuhiro Yamaguchi
University of California, Berkeley
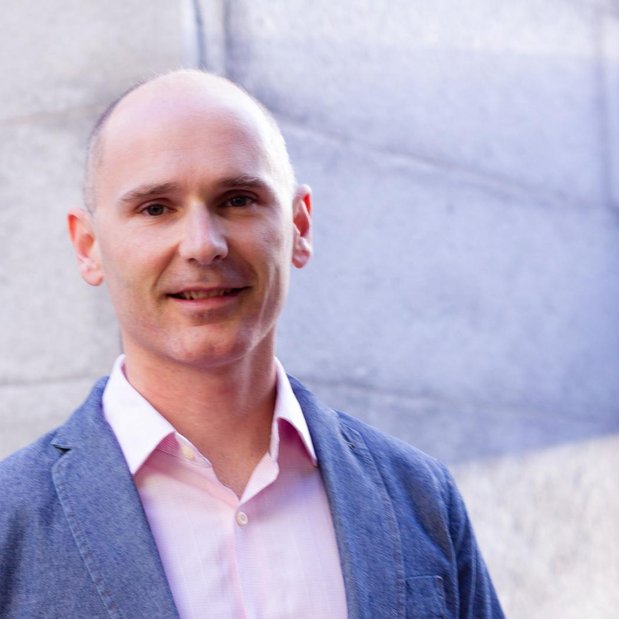
Alberto Todeschini
University of California, Berkeley
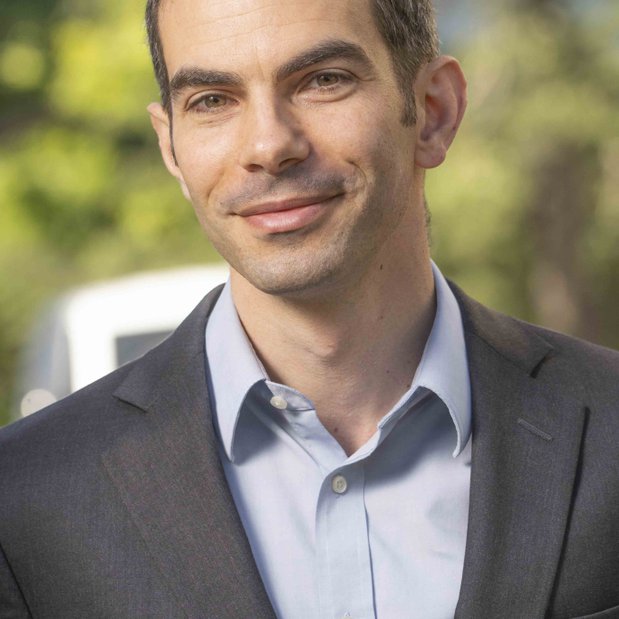
Joseph S. Shapiro
University of California, Berkeley