Tracking and Evaluation of Research, Development, and Demonstration Programs at the US Department of Energy
This report builds on a May 2023 RFF workshop that sourced experiences with and proposed best practices for developing the US Department of Energy's research, development, and demonstration (RD&D) capacity.
1. Introduction
With implementation of the Infrastructure Investment and Jobs Act (IIJA) and the Inflation Reduction Act (IRA), the US Department of Energy (DOE) will play a central role in driving the technological innovations needed to reach the Biden administration’s net-zero greenhouse gas emissions goal. However, it needs additional capacity in several areas, including how best to pick winners for the demonstration projects it will be funding. We held a workshop on this topic and developed a follow-on white paper (Bergman et al. 2023). Another area, the topic of this paper, is establishing the capacity to operationalize and institutionalize impact evaluations, which includes developing approaches for tracking data to support evidence-building and help evaluate DOE’s research, development, and demonstration (RD&D) programs. Strengthening evaluation capacity is important for two reasons: it permits DOE and other interested parties to evaluate the success of programs in advancing and, ultimately, commercializing technologies; and it provides input to the agency for adaptive learning to improve its guidance to applicants (in the Funding Opportunity Announcements [FOAs]), decision protocols, and data collection.
These two key pieces of legislation are not the only motivation for developing better program evaluations within DOE. The Evidence Act of 2018 US Congress, The Foundations for Evidence-Based Policymaking Act of 2018, HR 4174, Pul L 115-435, 115th Congress, signed into law in January 2019. aims to modernize federal government data collection and management processes to better inform policy decisions. It requires agencies to assess their evaluation practices and create a plan to develop evidence-building activities. The Office of Management and Budget (OMB) is charged with improving these activities and evaluation by providing guidance and resources to agencies and engaging with evaluation officers; at DOE, each individual office is responsible for both functions (DOE 2022), with program managers in charge of conducting evaluations while following program evaluation standards (OMB 2020).
This paper builds on a workshop held by Resources for the Future (RFF), informed by government publications and the academic literature, on evaluation and provides recommendations for building evaluation systems for DOE programs. Evaluation systems cover all the operational, organizational, and institutional elements that are needed, including human resources, organizational capacities, and evaluation practices. The report covers three topics: the state of program evaluation at DOE and in other relevant agencies, institutionalization of program evaluation within DOE, and characteristics of robust evaluation methods and their associated metrics and data needs. It closes with a series of recommendations.
2. Definition of Evaluation
The Evidence Act refers to evaluation as “an assessment using systematic data collection and analysis of one or more programs, policies, and organizations intended to assess their effectiveness and efficiency” (OMB 2020). DOE’s Office of Energy Efficiency and Renewable Energy (EERE) uses similar wording to define program evaluation (EERE n.d.a), “A systematic assessment using quantitative and/or qualitative data and analysis methods to answer specific questions about current or past programs, with the intent to assess their effectiveness and efficiency.” the main role being to produce knowledge to improve programs. Impact evaluations, which were the main focus of the workshop, are used to identify the causal effects of a specific program on a range of outcomes. They often involve randomized controlled trials or quasi-experimental approaches to estimate program impact through comparisons with a counterfactual situation without the program. Process evaluations, on the other hand, look at a program’s progress and monitor its implementation, with the intent of making process improvements (EERE n.d.a). DOE also does analyses which examine the characteristics of funded technologies and how they can integrate into energy systems and markets (EERE n.d.b), with the goal of producing knowledge for technology investment.
3. The Evaluation Landscape
Program tracking and evaluation are carried out by all agencies to varying degrees, partly based on legislative directives, such as the Evidence Act, specific programs, such as the Small Business Innovation and Research (SBIR) Program, Small Business Reauthorization Act of 2000, HR 5667. Pub. L. 106-554, 106th Congress, https://www.congress.gov/bill/106th-congress/house-bill/5667. the executive branch efforts, and an agency’s own commitment to success and accountability. This section discusses the evaluation landscape, including recent efforts, such as the Evidence Act and the policy response from DOE and, specifically, its Office of Energy Efficiency and Renewable Energy (EERE). We also discuss the work of peer agencies, such as the Department of Health and Human Services (HHS) and Environmental Protection Agency (EPA), which both have a long history of conducting evaluations. Finally, we extract lessons learned from these peer agencies and consider how DOE can work to institutionalize a culture of evaluation.
3.1. The Evidence Act and Administration Support
The Evidence Act US Congress, The Foundations for Evidence-Based Policymaking Act of 2018, HR 4174, Pul L 115-435, 115th Congress, signed into law in January 2019 is a recent installment in a series of legislative and executive branch efforts to promote program evaluation and evidence-building across the federal government (GAO 2021). Passed in 2019, the act is still being implemented. Each agency has to designate three senior officials in charge of promoting evaluation and data governance: an evaluation officer, a chief data officer, and a statistical official. All agencies must produce an annual evaluation plan and develop a strategic approach to evidence-building. Recognizing that capacity differs by agency, the act also requires publishing capacity assessments, which allow researchers and decisionmakers to take stock of the evaluation efforts and drive further research.
The current administration, through OMB (represented by Danielle Berman, who discussed OMB’s role in implementing this Title I of the Evidence Act at our workshop), has supported evidence-based policymaking by publishing guidance documents for agencies (OMB Circular No. A-11 Section 290, M-21-27, M-20-12, M-19-23, and M-22-12). These documents describe the value and purpose of agency-wide learning agendas and annual evaluation plans and encourage using the most rigorous methods appropriate for the evidence need. The Biden–Harris administration is especially dedicated to evidence-based policymaking through government-wide support to facilitate developing an evaluation culture, including professional development programs, technical assistance, and community-building efforts.
Based on comments by speakers and documents from the following agencies, we consider the evaluation efforts by the EERE because it is widely considered to be the agency’s leading office for evaluation. We also consider HHS and EPA evaluation efforts because their relatively well-developed culture could provide lessons for DOE. Finally, we discuss building an evaluation culture at DOE in the last part of this section.
3.2. DOE and EERE Efforts
In DOE’s fiscal year (FY) 2024 Evaluation Plan (DOE 2022), required under the Evidence Act and OMB guidelines, program evaluation is presented as key to managing a large portfolio of dissimilar programs and informing crucial decisions on planning and budget. However, DOE does not have an all-of-agency evaluation strategy. Rather, its plan focuses on processes and support at the agency level and delegates evaluation to functional offices and program managers.
DOE already uses a variety of evaluations to assess different aspects of its programs and offices. Although peer reviews (a form of process evaluation) have become common practice in most offices, we find this not true for systematic impact evaluations of RD&D programs’ effectiveness and efficiency, and agency-wide capacity is insufficient to conduct them. Between 2016 and 2021, 156 process evaluations (an average of 27 per year) and only 16 impact evaluations were completed (Dowd 2023). As new offices are created, such as the Office Clean Energy Demonstrations and Office of Manufacturing and Energy Supply Chains, it is important that programs are managed with future evaluations in mind.
Jeff Dowd, EERE’s lead program evaluator, described its efforts at the workshop. According to members of the evaluation community we contacted in other agencies, EERE has a relatively good record among federal agencies, particularly technology agencies, for tracking and evaluating projects and programs. According to EERE, key projects must be peer-reviewed every two years by an independent panel of experts. EERE also encourages technology offices to conduct impact evaluations assessing the causal effects of programs and outcomes against planned goals. However, offices have varied track records in conducting impact evaluations. EERE has been performing more impact evaluations over time with increasing rigor, including use of peer-reviewed methods. Most use quasi-experimental or nonexperimental methods, with some based on expert elicitations
Participants from across DOE discuss these efforts in monthly Evaluation Community of Practice meetings that share best practices among evaluators and program managers. According to Dowd, EERE also plans to increase its institutional capacity for impact evaluation by hiring new federal staff with relevant expertise and incentivizing technology offices to improve their capacity. EERE’s strategy also has an increased focus on embedding evaluation in program planning, execution, and decisionmaking by allocating more funds for impact evaluations, establishing new guidelines for quasi-experimental methods, and communicating the results. This strategy intends to build an evidence culture within EERE and could be spread to other parts of DOE. Although EERE has extensive data systems for tracking funding, project awards, and progress during the contract period, evaluators still face significant constraints when capturing outputs and outcomes, especially in the longer term, and these are critical to determine impacts. Establishing a data infrastructure to better support evaluation efforts is one element of EERE’s broader evaluation capacity-building strategy. Multiple efforts are ongoing to track programs’ outputs and outcomes from EERE’s investments; the data collected would help conduct and improve impact evaluations. Notable efforts include developing an evaluation data platform and tracking patent data and commercial technologies enabled by EERE investments.
3.2.1. EERE Evaluation Data Platform
Since 2022, EERE has been developing a new data infrastructure to directly support impact evaluation. The EERE Evaluation Data Platform Overview of Evaluation Data Platform, 2023, presentation available upon request from EERE. is a data repository and impact metrics reporting system that combines internal funding and project data (on awardees, contracts, project performance, etc.), external data (from non-DOE sources), and analytical tools to generate output, outcome, and impact metric reports and dashboards for EERE business users. It will also deliver project output and outcome data to commissioned evaluators for impact evaluations. The metrics will broaden the coverage of the EERE data systems by building on more than 300 data elements related to intellectual property, inventions and commercial technologies, attracted follow-on funding, energy savings and avoided emissions, and diversity and workforce, among other metrics. To the extent possible, these data collection and integration processes would be automated to better enable EERE to calculate impact metrics or provide data to evaluators to allow them to estimate impacts via appropriate experimental/quasi-experimental research designs. However, data from awardees outside of the contract period may still suffer from a low-response-rate bias that increases with time. By deploying this improved evaluation data infrastructure across the division, EERE hopes to be able to evaluate its results more efficiently and communicate the impacts that its funded RD&D is having across the US economy.
3.2.2. Tracking Commercial Technologies
For several decades, the Pacific Northwest National Lab (PNNL) has been tracking technologies for several EERE technology offices to provide EERE management with information about supported commercial and emerging technologies (Steele and Agyeman, 2021). A PNNL database documents the time to market of commercialized EERE-funded technologies by regularly soliciting projects’ points of contact and collecting data from companies’ websites, scientific publications, and interviewed technology developers after projects are completed, (Steele and Weakley, 2020). This effort, although long-standing and sometimes extensive has yet to be adopted by all EERE offices. The number of technologies tracked depends on the interest of EERE technology offices for information on their performance on commercialization metrics, which leads to some technologies being underrepresented in the database. The version we were able to access represented only a subset of the projects funded by the six technology offices PNNL has worked with: Advanced Manufacturing, Bioenergy Technologies, Buildings Technologies, Fuel Cell Technologies, Geothermal Technologies, Vehicles Technologies, and Wind Energy Technologies. For instance, in 2011, the geothermal office had a portfolio of more than 270 RD&D projects (DOE EERE 2011), but the version of the database we accessed only contained 15 commercial technologies. However, it should be noted that many projects are intended to advance research progress in the field and do not directly result in a commercial technology.
This type of data collection work, in some cases (e.g., when using surveys) can be very resource-intensive, and can suffer from a low-response-rate bias. Since the pandemic, the PNNL team has seen response rates dwindling from close to 100 percent to 75–80 percent when reaching out to projects’ points of contact for updates. This can be explained by difficulties in locating them due to retirements or changes in company staff or an increase in innovators’ confidential business information concerns. Besides, outside of the contracted period, data is provided voluntarily.
Although this data collection effort was not intended to provide data for impact evaluations, the derived commercialization metrics could help answer research questions about the effects of EERE’s innovation programs. The aim was to comply with the Government Performance and Results Act US Congress, Government Performance and Results Act of 1993, HR 826, Pul L 103-62, 103rd Congress, signed into law in August 1993. and the GPRA Modernization Act of 2010 US Congress, GPRA Modernization Act of 2010, HR 2142, Pul L 111-352, 111th Congress, signed into law in January 2011. and assess outcomes of EERE RD&D investments related to technology commercialization. Thus, this data has been leveraged to analyze EERE-funded technologies but not in the context of impact evaluations. One potential improvement to make the data more relevant would be to follow “losing” applicants (e.g., those that were selected but below the funding threshold), which is important for controlling for all the nonprogrammatic reasons for projects’ successes and failures. Another improvement would be to standardize the type of data collected on commercialization and market penetration across projects to facilitate aggregations and comparisons.
3.2.3. Tracking Patent Data
Patents are also targets of data collection efforts as a well-known but partial metric for technological innovation. In a study covering EERE-funded patents, researchers constructed a database containing all DOE grantees’ patents using tools such as the DOE Patents Database and iEdison, an interagency reporting system for recipients of federal funding agreements (DOE EERE 2022). Information on patents from DOE-funded projects can also be retrieved from the Government Interest section of the US Patent and Trademark Office (USPTO) and linked to project data. However, this data can require lengthy processing and verification to link patents with specific programs and funding contracts within DOE, which represents a barrier to a broader use of this information in impact evaluations.
3.3. Evaluation at HHS
Although no other agency is a perfect analogue to DOE, evaluation activities in other agencies might be relevant for clean energy technology innovation programs. For instance, evidence-building activities are well integrated in some agencies, such as HHS, which has an overarching evaluation strategy across programs carried out by multiple independent divisions and offices.
HHS, represented at our workshop by its evaluation officer, Susan Jenkins, has had a strong evaluation culture for decades, having established systemic agency-wide practices, committed to building adequate capacity, and implemented accountability to the evidence produced. Although HHS is decentralized and has varied operating divisions, similar to DOE’s organization, division liaisons attend the agency-level Evidence and Evaluation Council to ensure agency-wide coordination and feedback from the operational offices. This council predates the Evidence Act and includes senior evaluation staff and subject matter experts from each agency. The Office of the Assistant Secretary for Planning and Evaluation (ASPE) is responsible for understanding evaluation, research, and analysis efforts, collating the wide range of such efforts.
The evaluation officer has monthly meetings with strategic planning and performance management staff. Staff in all divisions also have ample training opportunities, ranging from gaining a basic understanding of the “how” and “why” for evaluation to conducting and interpreting the results. Nevertheless, Jenkins identified areas to improve the agency’s evaluation strategy, with some divisions still working to build up the necessary capacity (in both resources and workforce), improve data quality and access, and engage department leaders in the process.
HHS’s evaluation efforts under the Evidence Act encompass its whole organization. Operating divisions formulate individual evaluation plans based on a centralized template and guidance. These plans highlight priority questions, specific programs to be analyzed, data, and methods. Leadership within each of the divisions is responsible for approving the plans before they are compiled into the department-wide evaluation plan by ASPE.
In the department’s latest evaluation plan (HHS ASPE 2022), operating divisions provided examples to the Evidence and Evaluation Policy Council of significant evaluations planned across five priority areas: health care, public health, human services, research and evidence, and strategic management. These evaluations span all the divisions and are in differing stages of execution, yielding 23 evaluations, some in multiple priority areas.
Beyond the evaluation plan, the department is still implementing its learning agenda (HHS 2023), which is a four-year plan outlining the priorities and the methods and data required to answer them. It is also finalizing an update to its FY2023–2026 Capacity Assessment (HHS ASPE 2023), which assesses the “coverage, quality, methods, effectiveness, objectivity, scientific integrity, and balance” of the evaluation portfolio, and, more broadly, of their activities (OMB 2019).
The longstanding focus on evaluations at HHS, which began before the Evidence Act, provides several useful lessons that are relevant to DOE. Most importantly, robust department-wide evaluation work requires buy-in from all operating divisions, especially from agency leadership. Having a template and best practices from the department gives divisions the necessary guidance to craft their individual evaluation plans. Finally, agency-level coordination (i.e., ASPE) can be a valuable resource for taking stock of the agency’s evaluation efforts and identifying overlapping priority areas between divisions and evaluations that could address multiple priority areas.
3.4. Evaluation at EPA
Lessons about program evaluation and evidence-building activities can also be learned from EPA, which has some similar functions to DOE. At EPA, implementation of the Evidence Act is primarily through several priority areas (EPA 2022); the one most similar to DOE’s activities is evidence-building for the grantmaking process. EPA distributes over $4 billion into over 100 grant and other assistance programs each year, with 1,400 employees responsible for managing and tracking these. Similar to DOE, EPA’s system lacks comprehensive tracking, which inhibits its ability to evaluate program’s environmental outcomes.
At the workshop, Katherine Dawes, EPA’s acting evaluation officer, spoke about these challenges in data collection. Indeed, data can be challenging to standardize and consolidate across programs, making it difficult to track collective progress for the organization. In many cases, data may be provided in a variety of ways, including Word documents, PDFs, forms, or emails. Beyond data collection and the challenges in gathering confidential business information and personally identifiable information, the data quality itself may be poor, including issues such as missing or hard-to-find data and differing variable definitions. EPA has begun a three-year process to better understand the challenges with evaluating grants. The initial phase (Year 1) established a baseline to understand the existing grant award and reporting systems. In the second year, the Grant Commitments Workgroup examined specific practices and tools that could effectively track progress toward meeting workplan grant commitments.
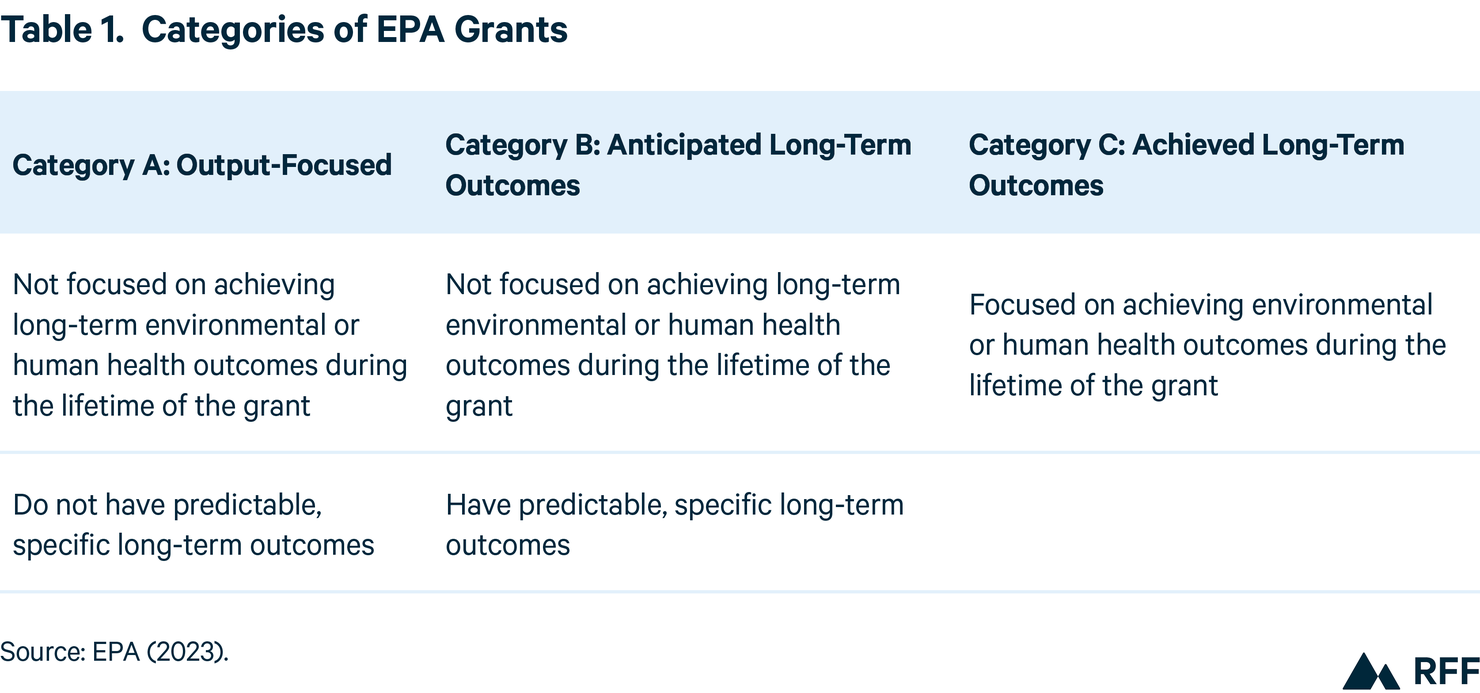
The Year 2 report (EPA 2023) has produced several recommendations, some of which may be applicable to DOE. For example, the workgroup suggested categorizing grant programs based on their anticipated results, such as a focus on achieving long-term environmental or human health outcomes or whether these outcomes are predictable (see Table 1). Based on this categorization, the agency can provide additional guidance or support for evaluation activities. Additionally, the Year 2 report recommends creating a flexible storage system to accommodate grants’ variety of data types and reporting schedules. Data types may be wide ranging, from quantitative (with standardized or nonstandardized metrics across grantees) to narrative (which may require an open field to tell a comprehensive story). The database should also allow for centralized document storage and be searchable across data metrics and document text.
In addition to data handling and individual grant programs, the Year 2 report also recommended greater guidance and templates from EPA headquarters on the administration’s priorities. This could range from setting the appropriate metrics for agency priorities to templates for data collection from grantees. Relatedly, the report also recommends greater internal communication. This would be most beneficial between administrative and technical staff to ensure quality and relevance of grantee-reported data and between HQ staff and employees who implement grants to improve understanding of how the data is used to communicate outcomes.
Beyond the recommendations themselves, creating a group at DOE similar to the EPA’s Grant Commitments Workgroup could be useful to improve data storage and reporting. The in-depth surveys and interviews conducted by the workgroup (described in its Years 1 and 2 reports) have helped EPA fine-tune its evaluation policies, creating greater cohesion across the agency. If DOE also seeks to build an evaluation culture across its offices, a similar department-wide initiative to understand inter- and intraoffice challenges could be helpful.
3.5. Institutionalization and Evaluation Culture
At the workshop, several ideas were suggested for institutionalizing evaluations at DOE (or other agencies) drawing from the agency’s own experiences and examples from other agencies, such as HHS and EPA, and guidance from the regulatory space. Joe Aldy (Harvard Kennedy School) presented a working paper on institutionalizing program evaluation by drawing lessons from regulatory agencies and past assessments of clean energy programs. Since 1981, they have been required to estimate benefits and costs of major regulatory proposals as part of their regulatory review process, with a majority of these from energy and environmental regulations. Policymakers can use the evidence generated to build a compelling argument for regulatory updates. Similarly, evaluations of tax incentives or spending programs, such as DOE’s RD&D grantmaking, can “enhance policymaker understanding of the most effective instruments for delivering on clean energy objectives” (Aldy 2022).
As highlighted, the DOE Evaluation Plan (DOE 2022) is limited in the breadth of its goals, as its four objectives mostly focus on the agency’s supporting activities and not the core programs. Yet, as highlighted by Aldy at the workshop, identifying priority outcomes to evaluate is one of the main steps to develop an evaluation strategy. Generally, DOE headquarters delegates evaluation to program managers. This does not build transparency in evaluation planning, which is needed because most of DOE’s technology offices do not have a good track record in evaluating program impact or making the analyses publicly available.
As discussed, DOE can learn from the practices established by other federal agencies, such as HHS and EPA. Both agencies have worked to build practices that standardize procedures for evaluations, and this has been strengthened by the requirement for a learning agenda and annual evaluation plan under the Evidence Act. Such requirements can guide an agency toward outlining the key priorities for evaluation and taking inventory of planned evaluations. For an organization with multiple operating divisions, such as HHS, a central council to encourage communication and information sharing has deepened the culture of learning, similarly to EERE’s Community of Practice. In outlining its evaluation priorities under the Evidence Act, EPA has initiated a multiyear plan to improve evidence-building and data collection around its grantmaking process, conducting organization-wide surveys and interviews. Not only are the recommendations from this process directly relevant to DOE, but a similar review process could identify and remediate the gaps in its own evaluation and data collection practices.
In addition to institutionalizing these practices, DOE could do more to promote a culture of evaluation, with a focus on retrospective analysis and iterative policymaking. Lessons can be learned from the practice of regulatory reviews in federal agencies (Aldy 2022). Regulatory agencies’ practices in this regard offer mixed results and a cautionary tale for DOE. Historically, they have been directed by the White House to review existing rules, but a “failure to meaningfully institutionalize retrospective review, build a culture of such review within agencies, and [allocate] appropriate monies” (Aldy 2022) have hampered their effectiveness. The best-laid plans can come to naught without a strong commitment from the top and middle management to see them through. The Evidence Act is a good start, as it endorses systematic evaluations and evidence-building activities, but history has indicated that such guidance may not be enough to overcome inertia within agencies. The administration’s budget proposal for 2024 (OMB 2023) affirms its commitment to evaluation, although no specific line item is dedicated to building evaluation capacity in DOE, where a large share of IIJA and IRA money will be spent. OMB supports the administration’s goal, but its authority is limited, so its guidance can only go so far in improving RD&D programs.
The barriers to developing evaluation capacities at DOE might be due to a lack of incentives. If the results of evaluations are not used to recommend or justify policy actions, process changes, or program improvements, program managers might see investing in them as a waste of their time and resources. Thus, it is important that DOE’s learning agenda and evaluation plan integrate the results into revisions and improvements. DOE leadership could spearhead communicating evaluations for “policymakers, stakeholders, the media, and the public,” which could create longstanding durable value and demand. Consolidating this information could provide support for annual budget requests to Congress and be a useful resource for funding on the scale of the IIJA and IRA once those spending provisions have sunset.
Another barrier might be the lack of qualified staff to conduct evaluations and initiate social experiments. One step that DOE could take to incentivize more evaluations of programs and processes at the agency level would be to create a position of chief economist, as suggested by Kyle Myers (Harvard Business School) in recommendations for the NIH (Myers 2023). They would have a quantitative background and oversee the overarching evaluation effort and social experiments for the different agency’s programs (we detail the importance of experimentation in the Research Design section). Having such a position at the agency or division level would create a gold standard for evaluations across the offices and programs. Most of these responsibilities could be carried out by the evaluation officer position, which is already required by the Evidence Act. Per OMB (2019) definition, they should have “substantive expertise in evaluation methods and practices” and “maintain principles of scientific integrity throughout the evaluation process.” The specific skillset in social science experiments that a chief economist would bring can be included under the purview of the evaluation officer if the employee has the appropriate expertise. For an organization of DOE’s size, qualified evaluators should be present throughout the agency rather than only at the top; those knowledgeable in social science methods are needed at the divisions level, where they can manage process and impact evaluations for the divisions’ programs. In addition, DOE could involve qualified social scientists to help build experiments and evaluation strategies for programs by leveraging the Intergovernmental Personnel Act (IPA) Mobility Program, as suggested by Myers in our workshop. The IPA allows federal agencies and other public bodies to hire from academia and other eligible institutions on a temporary basis.
Another significant barrier could be the cost and effort needed to access high-quality data for evidence-building activities. A prerequisite to RD&D program evaluation is access to data on applicants to measure outcomes and applicant characteristics. Yet, the FOA process is not designed to collect data for future impact evaluations. In addition, quantitative assessments are hampered by the lack of readily usable databases consolidating and organizing the data at the office or agency level and of available capacity to appropriately analyze the data and develop evidence-building activities. We discuss these data collection challenges in depth.
4. Evaluating Innovation: Methods, Metrics, and Data
In addition to considerations for operationalizing and institutionalizing evaluations at DOE, we must also consider how these evaluations will be conducted in practice. As in any evaluation, one needs to (i) define the unit of observation (the project); (ii) define questions to answer about the program and the outcomes that can answer those questions (metrics); (iii) collect data on the outcomes, such as technological uptake by purchasing firms, or on imperfect surrogates, such as the number of patents; (iv) consider factors (more metrics) that might affect the outcomes and collect data on them, such as grant characteristics (e.g., the size of award, timing, and other elements of the FOAs), grantee characteristics (e.g., revenues, profits, number of employees, private capital raised), and, if possible, similar characteristics of the losing applicants; and finally, (v) the research design (methods) to use to analyze the data. This section, which roughly aligns with the second session of our workshop, discusses the research design, metrics, and data necessary for program impact evaluation at DOE. Although we discuss these separately, they are all interdependent. Certain methods will require certain types of metrics, and the unavailability of metrics will require defining proxies that must be described by available data.
4.1. Research Design
The goal of impact evaluation is to understand and validate the effect of a policy (innovation grant programs) on a range of outcomes, including the behavior of actors affected by it. In the best-case scenario, to identify the causal effects, we want to compare two states of the world: a treated group affected by the program and a control group that has not been affected. According to our panelists, this evaluation design is best represented by randomized controlled trials (RCTs), which create the treated and control groups by introducing randomization into program implementation (through recipient selection, for instance) and exploits statistical differences between the two groups to identify the causal effects.
RCTs are not widely used to evaluate clean energy innovation programs, although they have been applied in similar programs in other fields. They have been used in medical research and economic development programs, which usually involve relatively small amounts of funding compared to some clean energy RD&D programs. However, as pointed out by Jacquelyn Pless, the design was successfully implemented in the context of a large urban infrastructure investment program in Mexico (McIntosh et al. 2018). This experiment, which was the largest of its kind, evaluated the impact of $68 million in investment randomly allocated across low-income urban communities on a range of critical outcomes. The researchers conducted an extensive household survey to collect data on both treated and control groups. The study was able to show a net improvement in some outcomes for treated neighborhoods. This large-scale RCT study is also a great example of researchers working closely with decisionmakers to include evaluation in their programs as an evidence-based policy tool to improve public spending efficiency.
That example focused on evaluating the impact on recipients, but RCTs can also be used to answer questions about program implementation and design. Components such as the composition of the selection committee, information shared, and structure of the funding mechanism can affect overall efficiency. Myers (2023) suggested a strategy to identify effective program design by leveraging controlled experiments for the National Institutes of Health.
Using controlled experiments, such as RCTs, in DOE evaluations might face barriers in the short term due to its novelty in the context of clean energy technologies, lack of funding to develop and apply them, or lack of agency or staff training regarding experimentation possibilities within existing programs. Controlled experiments are also not necessarily suitable to evaluate programs already in place. Thus, evaluators can turn to quasi-experimental approaches (natural experiments), where an element outside of the control of evaluators is used to create artificial treatment and control groups. This element creates randomness in how the program will affect different groups. DOE, for example, follows a selection process in which grant applications are scored by a small expert panel, and its ranking determines the applicants that are funded. By identifying those funded (the winners) with a score near the award cutoff and those not receiving funding (the losers) with a score just below the cutoff, the groups’ performance can be compared on a ceteris paribus basis, which is what Howell (2017, 2019) used to evaluate the impact of the SBIR program (see Box 1). Her research design relies on two key aspects: the availability of expert scores to rank applications and determine a group of similar applicants and the exogenous award cutoff, which creates randomness in funding allocation among the otherwise similarly scored grant applicants. This approach could be used more widely for program evaluation, conditioned on transparency on scoring, considering that grant selection through the traditional FOA process is similar within most DOE offices.
Since the IIJA bill, FOAs for technology demonstration projects often include a two-step process: a preselection phase, in which the candidate’s concept is “encouraged” or “discouraged” by DOE, and then the full application. Without the applications’ ranking to determine the two groups, selected applicants could be the treatment group and rejected encouraged applicants the control group. In FOA processes, reviewers could be asked to create scores for use in experiments, not just as input to the choice of winners.
Natural experiments in program evaluation can also arise from time or spatial discrepancies in implementation, which can create random treatment and control groups. For instance, Myers and Lanahan (2022) studied the spillover effects from the DOE SBIR program on research and development by exploiting the geographical differences caused by some states offering matching grants to successful local SBIR applicants, which creates random differences in funding amounts across states. To remove the potential endogeneity bias from DOE not making random SBIR investments, the overall effect of funding on patenting activity is identified using the effect of the randomly distributed matching grants on patenting. This natural experiment confirmed that the program generates large technological spillovers. DOE could leverage this kind of evaluation design when local or state policies, regulation, or other specificities create random differences in how a program impacts innovators. Such state-level variations could arise from access to certain infrastructure or the local permitting process.
Box 1. Small Business Innovation and Research Program Evaluation
In the context of a Congress-required evaluation by the National Academy of Sciences (NAS), several studies have been conducted on the SBIR program, such as Howell (2017, 2019), which is included in the follow-up NAS study focusing on the program (NAS, 2020).
SBIR is a cross-agency grant program designed to stimulate innovations from small businesses and help commercialize them. DOE has participated in it since 1982 and releases a multi-topic FOA twice a year to select new awardees. The Small Businesses Administration centrally collects the amount and names of recipients of all attributed awards. As recipients are mainly small firms, finding data about innovation outcomes, such as their patenting and business activities, is fairly easy through public and private sources.
Howell exploited the ranking of SBIR applications to compare the innovation and business performances of start-ups above and below the award cutoff to determine the program’s impacts. The innovation performance is measured by the patent count associated with each start-up, and the business performance includes the amount of venture capital raised, firm acquisitions, and firm survival. Patent data comes from DOE internal databases and public sources; business-related data is from proprietary databases. The ranking of grant applications, although crucial to designing this evaluation, is not publicly available for DOE’s SBIR or other competitive grant programs, and it can be very difficult to get permission to gain access to program data (NAS, 2020).
Howell found evidence of the innovation benefits of the program, with a Phase 1 award increasing a firm’s cite-weighted patents by at least 30 percent and chances of receiving venture capital by more than 10 percent.
Unfortunately, natural experiments are not always easy to discover or use for evaluation. Myers suggested that collaboration between social science researchers and experts from DOE who are knowledgeable about the inner workings of innovation programs can help find where randomness exists and how it can be incorporated into the evaluation strategy. Similarly, Pless et al. (2020) advocated that collaboration between researchers and funding agencies would help find situations where RCTs or quasi-experiments can be used. Working with state level or local authorities might also help discover regional discontinuities and local characteristics that will affect implementation.
It might be difficult to find a control group in various settings. Collecting data on a control group can be difficult outside of an RCT framework. For instance, tracking and collecting information on “losers” (the unsuccessful applicants) can be burdensome because they are not under contract with the funding agency and thus have no obligation to provide data or reporting. We cover this issue extensively in the data section of this report.
Studying only the treated group will not provide as meaningful and significant evaluation results but could still be valuable. According to Pless, one way to estimate causal effects without data on “losers” might be to study the policy interaction between different types of innovation programs. Pless (2022) estimated the effect of grant funding and tax credit interactions on RD&D activity in the United Kingdom. The study used a difference-in-discontinuities design that estimates if a change in the tax credit amount will impact the change in RD&D expenditures around the funding threshold for the grant program. Pless found that the two policies are complementary for small firms but seem to be substitutes for larger firms. This type of research design might be applicable when looking at certain technologies supported by DOE that benefit from both direct grant funding and tax credits. For instance, carbon capture, use, and storage technologies are targeted by both grant funding for RD&D projects within the IIJA and the 45Q tax credit, which was made more generous in 2022 as part of the IRA.
4.2. Metrics
Measuring progress toward DOE’s multiple objectives is a complex task. One issue is that they may be too vague to serve as metrics or have no data available, so proxy measures can be used. These are observable and quantifiable metrics correlated to the underlying intangible metric. For instance, the main goal of DOE’s RD&D grant programs is to support innovation. However, as “innovation” refers to a process, multiple metrics can be used to capture the different characterizations of the underlying objective, which include knowledge creation but also diffusion in the market depending on where the technology is on the innovation scale. These are often proxied by patenting activity and by the take-up of new technologies, the time to take up, and creation of new companies or product lines, respectively.
In his presentation, Adam Jaffe (Brandeis University) said that a good proxy metric should have a high signal to noise ratio, meaning that it should reflect as closely as possible the real value of the metric. Evaluators also should be careful of other measurement problems that can arise, such as potential bias and correlations between the chosen metric for a goal and the other goals. Chosen metrics should also have a stable relationship over time with the true objective measured. Finally, evaluators should be careful of potential for metrics manipulation.
Jaffe warns that using indicators may lead to adverse policy effects if achieving good results in the proxy metric rather than the actual objective becomes the goal. For instance, measuring innovation progress only through patents publications gives a biased picture of a program’s progress. Patents published represent a specific approach to knowledge creation but not necessarily the later stages of innovation, which involves scaling technologies to a commercial level and market diffusion. These last steps are considered a “second Valley of Death” for energy technologies: due to the important risks and costs entailed by industrial demonstrations, innovators struggle to attract sufficient private funding to scale their projects (Hart 2020).
Another challenge in measuring the effects of innovation programs highlighted by Jaffe is the typical lag between the policy intervention and realizing the desired outcomes. Although DOE RD&D grant contracts generally last two years (with one notable exception being the new funding for demonstrations authorized by the IIJA and IRA), their effects might not be seen until several years after they end. According to research commissioned by EERE, among patents that resulted from EERE grants and assistance programs, innovators averaged more than four years after the start of the project to apply for a patent and more than seven years for the patent to be published (DOE EERE 2022). In addition, in the energy sector, the development time is usually longer due to the large-scale demonstrations needed to ensure market readiness (Gaddy et al. 2017).
To address this lag issue, evaluators can use intermediate outcomes as an indicator of the progress toward the long-term outcome or the likelihood that it is realized. To represent good metrics, these intermediate outcomes should be explicitly linked to the underlying objectives. Regarding innovation, the level of private investment raised in a certain low-carbon technology can constitute an intermediate outcome that informs the likelihood of new knowledge being created or new products being developed in the coming years.
Evaluators should also be mindful of the impact of extreme observations on metrics. If a given RD&D grant program has a relatively small number of projects, one extreme outcome can greatly influence the measurement and have a significant impact on the lessons learned from a program. For instance, the Solyndra failure in 2011 was viewed as a failure of the Loan Program Office model as a whole and led to a loss of trust in the program for several years afterward, but its total portfolio losses stayed fairly low.
Researchers at RFF have identified useful metrics to select demonstration projects that capture the associated costs, benefits, and other considerations relevant to the decisionmaking process (Bergman et al. 2023) that can also be used for evaluation. A central metric is new benefits of a program, with costs covering the governmental funding and potential negative environmental or social impacts. One obvious benefit is the reduction in polluting emissions, which can be monetized through the social cost of carbon or $/ton estimates available for other pollutants (e.g., the value of the economic damages that result from emitting one additional ton of carbon dioxide into the atmosphere) (RFF n.d.). Another tricky metric is job creation, as it is hard to know if a new job is created or the result of shifting jobs around.
For programs targeting technologies that are higher on the Technology Readiness Level (TRL) scale, metrics could also focus on the competitiveness with incumbent technologies or products. Innovations in the energy sector are competing with well-established carbon-intensive incumbent technologies and products whose prices are generally far lower than their less carbon-intensive counterparts. Hence, evaluating the capacity of grant programs to reduce the costs of innovative technologies will inform progress toward commercialization. The manufacturability of innovative technologies is another key aspect to inform progress toward this goal, especially for higher TRL technologies (Bossink 2020), and can be measured by looking at economies of scale and, ultimately, costs.
4.3. Data for Evaluation
The availability of high-quality output data, outcome metrics, and variables that could explain outcomes is a prerequisite for any robust evaluation, regardless of the methods chosen. Some of the data can be qualitative, some quantitative. The data sources are also wide ranging, which has consequences for ease of access. For instance, patent data is public and easily accessible on USPTO, whereas data on technology costs might be viewed as sensitive business information, which is likely to stay private, unless disclosure terms are contained in the FOAs governing the acceptability of an application. In this section, we highlight key friction points regarding data accessibility, collection, and management for program evaluation.
DOE’s offices are already producing and collecting some data for use in evaluations through the grant application process and the potential reporting requirements during the funding agreements (e.g., applicants’ budget and goals, project awardee funding, milestone achievements, and outputs by projects), although they lack a systematic and standardized approach to collecting output and outcome data for funded projects. Program managers in charge of selecting grantees collect and produce data on applicants to inform funding decisions, which can be used to create comparison groups based on applicants’ characteristics or reviewers’ scores (see Box 1 for an example). In addition, internal post-award tracking efforts (such as the iEdison database on federally funded patents or PNNL commercialization database) can be a source of data on recipients’ activities, especially after the end of the funding contract. However, these efforts are currently not widely used for impact evaluation and would benefit from being more extensive and standardized across offices. For example, for commercialization, additional information could include company size, workforce, and geographic location). The EERE Evaluation Data Platform will help improve and standardize data collection. In addition, FOAs from all technology offices could more consistently require applicants to report some information before, during, and after the funding contract. These efforts focusing on recipients may not provide the data necessary to establish a baseline or a comparison group to measure the effects of a program, which is key for evaluation.
Reporting requirements in funding contracts should include some provision addressing confidential business information to facilitate grantee disclosure. Due to the nature of clean energy innovation programs, recipients’ outcome information can be hard to come by due to a desire for secrecy. Many applicants do not want other applicants, shareholders, ratings agencies, and others to know of their plans. Sometimes, asymmetric information can be beneficial for applicants that might prefer not to reveal their true performance to funders in the hope of receiving more funding. Sometimes, it may be to avoid the embarrassment of losing, the possibilities of giving away trade secrets, or giving an edge to competitors. DOE’s Clean Hydrogen Hubs (H2Hubs) program is one telling example; the competition between and within hubs has prevented publishing detailed plans that are considered sensitive business information (RBN Energy, 2023). The desire for secrecy may also be due to sheer inertia favoring past processes or applicants finding reporting requirements burdensome, especially if they are a smaller organization or a loser.
DOE FOAs for grant funding all follow a similar selection process where applications submitted are scored based on broad categories: technical/scientific merit, proposed execution plan, and applicant’s team and resources. As required by the IIJA and IRA, Community Benefit Plans are now also included and account for 15–20 percent of the score. The information collected by program managers on both selected and rejected applicants can help establish baselines and comparisons for evaluations.
Then, once selected and funded, projects undergo peer reviews, at least biannually for EERE, where the grantees present their project’s progress to a panel of experts, which scores each one based on its approach, accomplishments compared to its initial targets, extent of its efforts, relevance to DOE programs, and proposed work. Go/no-go targets, delays, extensions, patent applications mentioned in peer reviews are information that can be collected to build metrics.
FOAs are not designed to collect data after the end of the funding contract. Although FOAs under IIJA programs have reporting requirements, these are not specific in terms of the type of data, and their binding nature is not specified. For instance, the Regional H2Hubs FOA specifies that “DOE will require project, environmental, technical, financial, operational, and socio-economic data collection and reporting for the H2Hubs,” whereas grantees are only “encouraged to voluntarily provide operating performance data beyond the period of performance for the award,” which is in no way binding for funded projects (OCED 2022).
Regarding the lack of precision in reporting requirements, Aldy (2022) advised that the data collection requirements should minimize the need for interpretation by grantees. To ensure that the data collected is standardized, reporting should follow detailed guidelines, including clear definitions of the data expected and potential calculation methods. For instance, estimating job creation, such as in demonstrations programs’ Community Benefits Plans, is a difficult task because several definitions can apply even in the literature, which can lead to misinterpretations, inconsistent data collection, and biases in evaluations.
Information reported by recipients is only one piece of the data collection puzzle. Useful data is also collected by other government agencies or through private initiatives. Financial information, such as revenues, private investment raised, or acquisitions, are collected by private intelligence providers, such as Crunchbase, as used by Howell (2017). Numerous entities are already gathering large quantity of data relevant to clean energy innovation programs: the Energy Information Administration on energy use and efficiency, Bureau of the Census on businesses, employment, and income at a very granular level, EPA on emissions at the facility level and other types of pollution, and Bureau of Labor Statistics on employment (Aldy 2022). USPTO also publishes data on patenting activity. These sources can provide information on nonrecipients, but it might be difficult to obtain granular data due to privacy concerns. However, these data sources can also help with evaluating programs community benefits, which are central to DOE’s environmental justice goals. For instance, evaluators could estimate the local economic, social, and environmental effects of clean energy demonstration projects.
Access to these external datasets could be helped through interagency partnerships and standardizing pathways and naming systems between datasets. For instance, to facilitate tracking patent applications funded by public grants, USPTO includes a Government Interests section in patent documentation where the applicants should acknowledge the government contract number through which they received funding. This linkage can complement the iEdison database, which collects the data required by the Bayh-Dole Act and the related regulations. However, both data sources are subject to underreporting bias (Onken et al. 2019). Aldy (2022) mentioned that an initiative such as the Census Data Linkage Infrastructure could be leveraged to match different agency datasets relevant to clean energy programs.
Relevant data to measure innovation and commercialization can be obtained through private data providers specializing in business intelligence, which includes sources such as Crunchbase, OpenCorporates, and Cleantech Group I3; researchers often use them to survey business activity through the amount of funding raised, status (firm survival rate), and especially small businesses (Dalle et al. 2017; Howell 2017; Onken et al. 2019). Such databases can provide detailed information on companies, related individuals, venture capital activity, and acquisitions, all of which can inform innovation and commercialization metrics. Business intelligence data can often be more reliable than similar data collected through surveys, which can suffer from low-response rate and sample-selection biases (Onken et al. 2019). In addition, Onken et al. (2019) showed that surveys can be very costly compared to access to these extensive proprietary databases.
5. Recommendations
We conclude with two sets of recommendations for DOE drawn from input from the workshop and our research: devoted to boosting evaluation, based on the material in Sections 3 and 4, and implementing evaluation, based on the material in Section 5.
5.1. Boosting Evaluation at DOE
DOE leadership should be actively involved in establishing an evaluation culture and institutionalizing practices within divisions.
Any plan to promote or institutionalize a culture will require buy-in from leadership beyond stating that evaluations should be conducted. Doing so without a plan or culture can be counterproductive.
DOE should develop an all-of-agency evaluation strategy with the division leaders, similar to the practices at HHS, rather than delegating to individual divisions and program managers. This strategy should stem from a collaboration between experienced qualified evaluation specialists from both the agency’s front office and the divisions.
ASPE at HHS provides essential guidelines, support, and templates for divisions to craft their evaluation plans, which it aggregates into an agency-wide plan. This unified effort, which garners buy-in from division leaders, could serve as a useful example for how DOE can build its own iterative and retrospective evaluation culture. Most importantly, it should be consistent across the agency’s operating divisions and involve a strong collaboration between the DOE’s evaluation officer and chief division evaluators.
DOE should perform an overarching review of its data collection and grant tracking efforts to help identify key areas for improvement and coordination across divisions.
EPA’s three-year review of its grantmaking data and metrics can serve as a blueprint for a similar effort at DOE. This would be useful due to the patchwork nature of data collection at DOE and varying levels of emphasis on this subject across divisions. Similar to EPA, DOE could establish a workgroup that can survey and interview stakeholders across the organization.
DOE should focus on iterative, retrospective evaluation while avoiding the pitfalls of similar efforts in the regulatory space.
The Evidence Act provides a useful high-level endorsement for evaluation but has only limited authority for shaping RD&D programs. Additional work and adequate resources are necessary to institutionalize retrospective evaluations or reviews and a culture for evaluations. Leadership should emphasize the importance of this work, including its role in annual budget requests to Congress and as a communication tool for stakeholders and the public.
DOE should invest in developing their team of qualified specialists for impact and process evaluation.
To ensure that evaluations are performed more systematically across divisions, qualified evaluation specialists should be dedicated to the task. They should have a range of essential technical skills and expertise, including developing evaluation questions, developing and appropriately using experimental and quasi-experimental research designs, and applying social sciences methods. Agency resources should be allocated to hiring federal and contractor staff with these characteristics or further strengthening these capacities in-house.
5.2. Implementing Evaluation at DOE
DOE should define objectives and outcome metrics and identify outcome proxies up-front.
Program managers and agency leadership, perhaps in collaboration with the OMB, need to define clear program goals and translate those into specific outcome metrics. To explain variations across outcomes for a set of projects (or even across a set of programs), additional “input” variables need to be defined, data sought, and proxies identified if data are unavailable from the onset. These variables can be grouped into program characteristics, applicant characteristics (including losers, if possible), application characteristics, etc. Sometimes, identifying intermediate outcomes can provide short-term indications of program success. Defining metrics and seeking data for evaluating Community Benefit Plans presents its own set of challenges that calls for DOE-wide dedicated and extensive efforts.
DOE should develop and implement data collection and management processes up-front (data on outcomes, outputs, and inputs).
In FOAs developed in response to IIJA and IRA programs, DOE highlights that they will collect data on the technological, economic, social, and environmental aspects of the funded projects, and hopefully these requirements will be specified during the award negotiation’s period. However, DOE could systematize and better specify these data collection requirements and include post-award data collection as a binding requirement to better prepare for future evaluations. Designing FOAs that make provision of specific data by bidders a condition for their application being reviewed for funding can address many of the issues that stymie high-quality evaluations.
Of course, potential applicants may decline to apply unless their confidential business information is protected. Research on how this can be done to satisfy applicants, meet evaluation needs and work within the legal system is urgently needed. Another suggestion is to develop a working knowledge of databases and data series available throughout the federal government and private sector to aid in the data collection task.
The Evaluation Data Platform effort funded by EERE is promising for its data collection and management and should help conduct successful evaluations. Developing and implementing this tool should be closely followed by those in DOE who are interested in evaluation; if proven successful, it could be extended to other divisions or the entire agency.
DOE should make data available to outside groups for doing non-DOE sponsored evaluations.
The large dollar amounts placed into new programs with passage of the IIJA and IRA raise transparency concerns from taxpayers and stakeholders interested in these programs, which could be alleviated, in part, by making data available to the public and/or to outside groups for accountability and evaluation purposes (with confidential business information protected).
DOE should develop an evaluation database.
A secure system for finding, storing, and retrieving information linked to funding opportunities and collected for the evaluation is needed. Ideally, it would follow applicants for enough time that the full benefit of the grant is realized. Legal structures must be put into place to protect confidential business information, while ensuring access for evaluators with appropriate protections. The Evaluation Data Platform is a big step in the right direction, but it will likely suffer from a low-response-rate bias from awardees when collecting information after the contract period or concerning confidential business information.
DOE should develop methods for evaluating outcomes.
The gold standard of impact evaluation is to compare program beneficiaries with a control group. Comparing the outcomes of grant winners to losers with close scores (similar to the SBIR example in Box 1) is a good application because it isolates the effects of the grant compared to all other possible effects. Searching for random variation in program design or implementation could identify other natural experiments. If the gold standard is unattainable, less rigorous but still very useful approaches to evaluation exist, such as the use of random variation in program design or implementation, as detailed. Close consultation among government and academic evaluators can aid in developing and implementing evaluation methods.